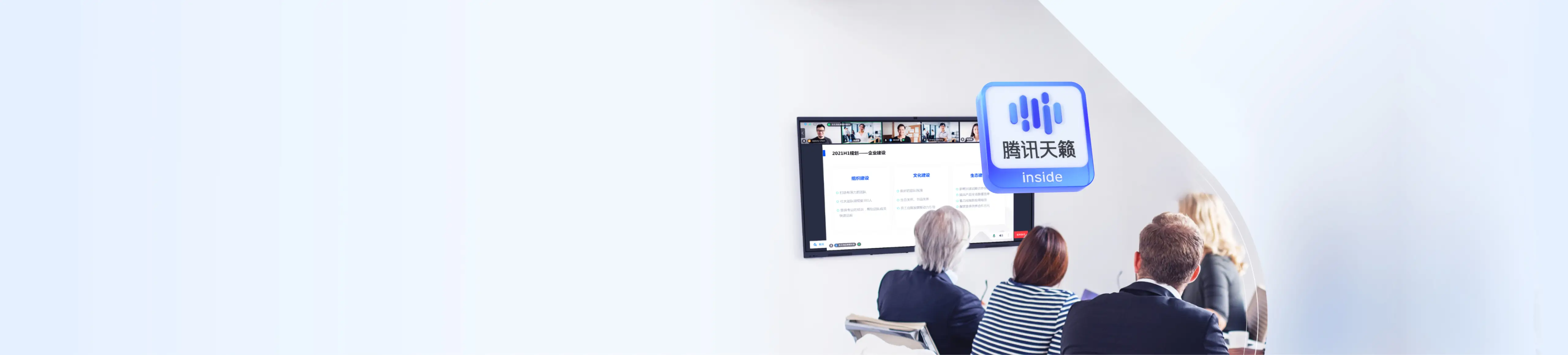
论文研究
前沿技术探索
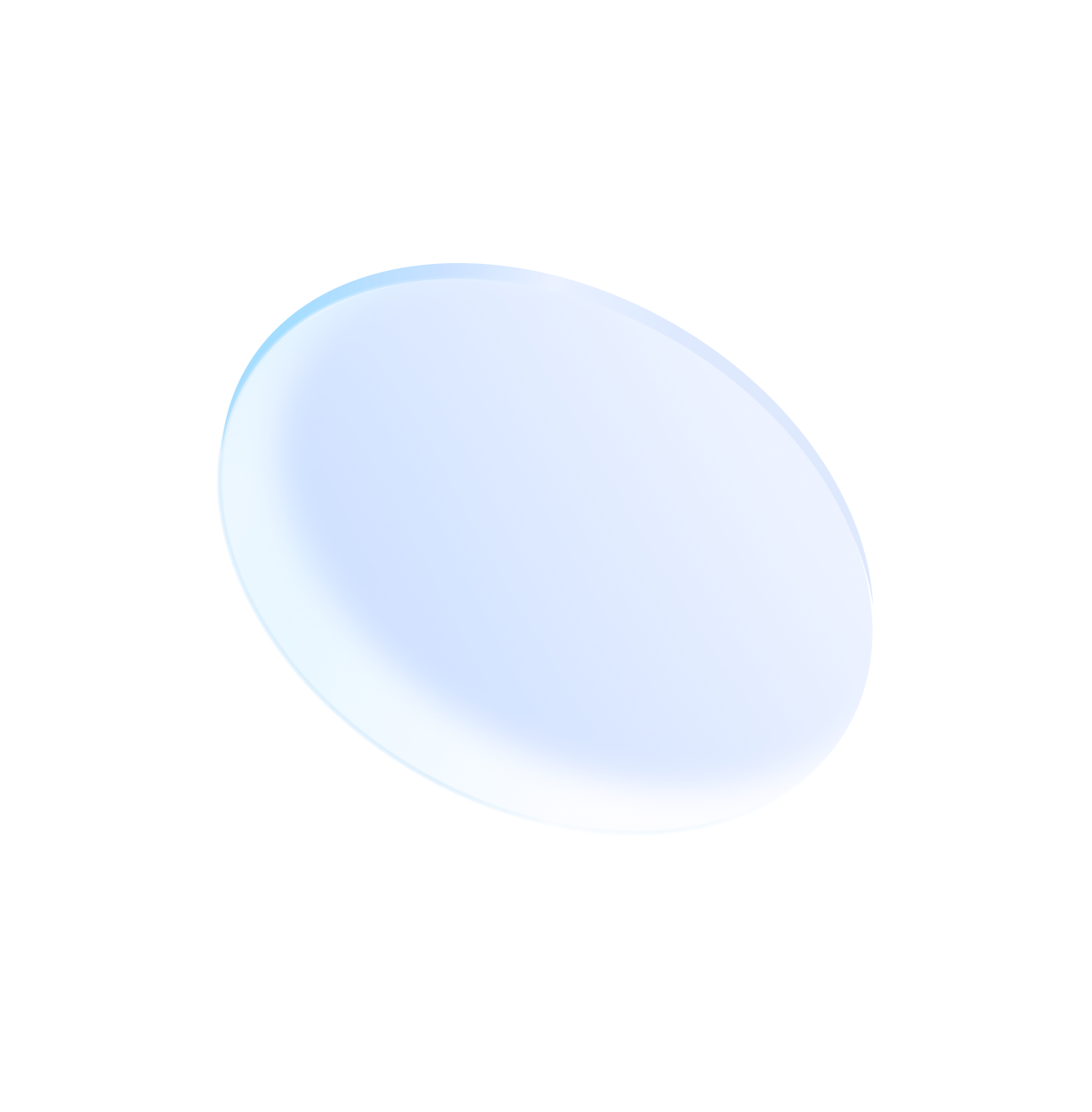
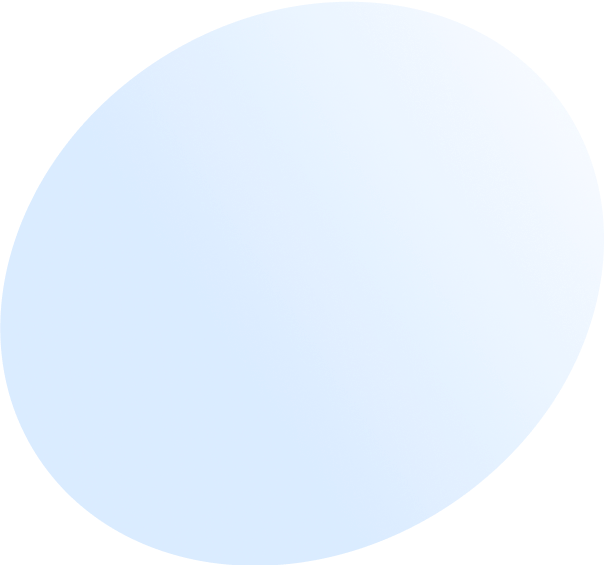
Gesper: A Unified Framework for General Speech Restoration
Jun Chen; Yupeng Shi; Wenzhe Liu; Wei Rao; Shulin He; Andong Li; Yannan Wang; Zhiyong Wu; Shidong Shang; Chengshi Zheng
This paper describes the legends-tencent team’s real-time General Speech Restoration (Gesper) system submitted to the ICASSP 2023 Speech Signal Improvement (SSI) Challenge. This newly proposed system is a two-stage architecture, in which the speech restoration is performed, and then followed by speech enhancement. We propose a complex spectral mapping-based generative adversarial network (CSM-GAN) as the speech restoration module for the first time. For noise suppression and dereverberation, the enhancement module is presented with fullband-wideband parallel processing. On the blind test set of ICASSP 2023 SSI Challenge, the proposed Gesper system, which satisfies the real-time condition, achieves 3.27 P.804 overall mean opinion score (MOS) and 3.35 P.835 overall MOS, ranked 1st in both track 1 and track 2.
2023年6月4日
阅读全文TEA-PSE 3.0: Tencent-Ethereal-Audio-Lab Personalized Speech Enhancement System For ICASSP 2023 Dns-Challenge
Yukai Ju; Jun Chen; Shimin Zhang; Shulin He; Wei Rao; Weixin Zhu; Yannan Wang; Tao Yu; Shidong Shang;
This paper introduces the Unbeatable Team’s submission to the ICASSP 2023 Deep Noise Suppression (DNS) Challenge. We expand our previous work, TEA-PSE, to its upgraded version – TEA-PSE 3.0. Specifically, TEA-PSE 3.0 incorporates a residual LSTM after squeezed temporal convolution network (S-TCN) to enhance sequence modeling capabilities. Additionally, the local-global representation (LGR) structure is introduced to boost speaker information extraction, and multi-STFT resolution loss is used to effectively capture the time-frequency characteristics of the speech signals. Moreover, retraining methods are employed based on the freeze training strategy to fine-tune the system. According to the official results, TEA-PSE 3.0 ranks 1st in both ICASSP 2023 DNS-Challenge track 1 and track 2.
2023年6月4日
阅读全文TEA-PSE: Tencent-Ethereal-Audio-Lab Personalized Speech Enhancement System for ICASSP 2022 DNS Challenge
Yukai Ju; Wei Rao; Xiaopeng Yan; Yihui Fu; Shubo Lv; Luyao Cheng; Yannan Wang; Lei Xie; Shidong Shang;
This paper describes Tencent Ethereal Audio Lab – Northwestern Polytechnical University personalized speech enhancement (TEA-PSE) system submitted to track 2 of the ICASSP 2022 Deep Noise Suppression (DNS) challenge. Our system specifically combines the dual-stage network which is a superior real-time speech enhancement framework with the ECAPA-TDNN speaker embedding network which achieves state-of-the-art performance in speaker verification. The dual-stage network aims to decouple the primal speech enhancement problem into multiple easier sub-problems. Specifically, in stage 1, only the magnitude of the target speech is estimated, which is incorporated with the noisy phase to obtain a coarse complex spectrum estimation. To facilitate the formal estimation, in stage 2, an auxiliary network serves as a post-processing module, where residual noise and interfering speech are further suppressed and the phase information is effectively modified. With the asymmetric loss function to penalize over-suppression, more target speech is preserved, which is helpful for both speech recognition performance and subjective sense of hearing. Our system reaches 3.97 in overall audio quality (OVRL) MOS and 0.69 in word accuracy (WAcc) on the blind test set of the challenge, which outperforms the DNS baseline by 0.57 OVRL and ranks 1st in track 2.
2022年4月27日
阅读全文A Maximum Likelihood Approach to Masking-based Speech Enhancement Using Deep Neural Network
Qing Wang; Jun Du; Li Chai; Li-Rong Dai; Chin-Hui Lee
The minimum mean squared error (MMSE) is usually adopted as the training criterion for speech enhancement based on deep neural network (DNN). In this study, we propose a probabilistic learning framework to optimize the DNN parameter for masking-based speech enhancement. Ideal ratio mask (IRM) is used as the learning target and its prediction error vector at the DNN output is modeled to follow statistically independent generalized Gaussian distribution (GGD). Accordingly, we present a maximum likelihood (ML) approach to DNN parameter optimization. We analyze and discuss the effect of shape parameter of GGD on noise reduction and speech preservation. Experimental results on the TIMIT corpus show the proposed ML-based learning approach can achieve consistent improvements over MMSE-based DNN learning on all evaluation metrics. Less speech distortion is observed in ML-based approach especially for high frequency units than MMSE-based approach.
2018年11月26日
阅读全文A maximum likelihood approach to deep neural network based speech dereverberation
Xin Wang; Jun Du; Yannan Wang
Recently, deep neural network (DNN) based speech dereverberation becomes popular with a standard minimum mean squared error (MMSE) criterion for learning the parameters. In this study, a probabilistic learning framework to estimate the DNN parameters for single-channel speech dereverberation is proposed. First, the statistical analysis shows that the prediction error vector at the DNN output well follows a unimodal density for each log-power spectral component. Accordingly, we present a maximum likelihood (ML) approach to DNN parameter learning by charactering the prediction error vector as a multivariate Gaussian density with a zero mean vector and an unknown co- variance matrix. Our experiments demonstrate that the proposed ML-based DNN learning can achieve a better generalization capability than MMSE-based DNN learning. And all the object measures of speech quality and intelligibility are consistently improved.
2017年12月12日
阅读全文Gaussian density guided deep neural network for single-channel speech enhancementn
Li Chai; Jun Du; Yannan Wang
Recently, the minimum mean squared error (MMSE) has been a benchmark of optimization criterion for deep neural network (DNN) based speech enhancement. In this study, a probabilistic learning framework to estimate the DNN parameters for single-channel speech enhancement is proposed. First, the statistical analysis shows that the prediction error vector at the DNN output well follows a unimodal density for each log-power spectral component. Accordingly, we present a maximum likelihood (ML) approach to DNN parameter learning by charactering the prediction error vector as a multivariate Gaussian density with a zero mean vector and an unknown covariance matrix. It is demonstrated that the proposed learning approach can achieve a better generalization capability than MMSE-based DNN learning for unseen noise types, which can significantly reduce the speech distortions in low SNR environments.
2017年9月25日
阅读全文A Maximum Likelihood Approach to Deep Neural Network Based Nonlinear Spectral Mapping for Single-Channel Speech Separation
Yannan Wang; Jun Du; Li-Rong Dai; Chin-Hui Lee
In contrast to the conventional minimum mean squared error (MMSE) training criterion for nonlinear spectral mapping based on deep neural networks (DNNs), we propose a probabilistic learning framework to estimate the DNN parameters for singlechannel speech separation. A statistical analysis of the prediction error vector at the DNN output reveals that it follows a unimodal density for each log power spectral component. By characterizing the prediction error vector as a multivariate Gaussian density with zero mean vector and an unknown covariance matrix, we present a maximum likelihood (ML) approach to DNN parameter learning. Our experiments on the Speech Separation Challenge (SSC) corpus show that the proposed learning approach can achieve a better generalization capability and a faster convergence than MMSE-based DNN learning. Furthermore, we demonstrate that the ML-trained DNN consistently outperforms MMSE-trained DNN in all the objective measures of speech quality and intelligibility in single-channel speech separation
2017年8月20日
阅读全文A Gender Mixture Detection Approach to Unsupervised Single-Channel Speech Separation Based on Deep Neural Networks
Yannan Wang; Jun Du; Li-Rong Dai; Chin-Hui Lee
We propose an unsupervised speech separation framework for mixtures of two unseen speakers in a single-channel setting based on deep neural networks (DNNs). We rely on a key assumption that two speakers could be well segregated if they are not too similar to each other. A dissimilarity measure between two speakers is first proposed to characterize the separation ability between competing speakers. We then show that speakers with the same or different genders can often be separated if two speaker clusters, with large enough distances between them, for each gender group could be established, resulting in four speaker clusters. Next, a DNN-based gender mixture detection algorithm is proposed to determine whether the two speakers in the mixture are females, males, or from different genders. This detector is based on a newly proposed DNN architecture with four outputs, two of them representing the female speaker clusters and the other two characterizing the male groups. Finally, we propose to construct three independent speech separation DNN systems, one for each of the female-female, male-male, and female-male mixture situations. Each DNN gives dual outputs, one representing the target speaker group and the other characterizing the interfering speaker cluster. Trained and tested on the speech separation challenge corpus, our experimental results indicate that the proposed DNN-based approach achieves large performance gains over the state-of-the-art unsupervised techniques without using any specific knowledge about the mixed target and interfering speakers being segregated.
2017年5月2日
阅读全文